How AI-powered brain implants are helping an ALS patient communicate
The Stanford research involves an algorithm that interprets brain signals and then tries to translate them into words.
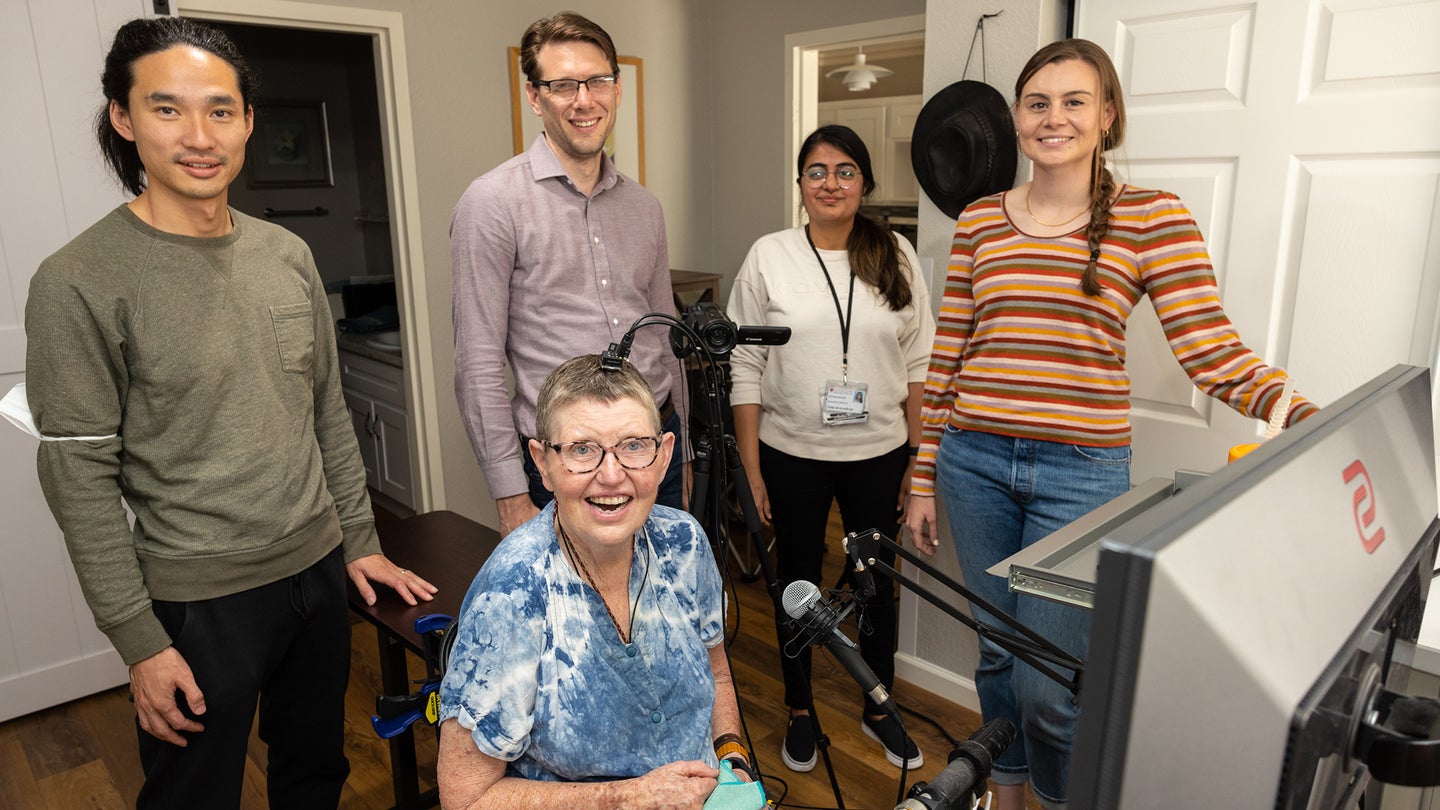
Nearly a century after German neurologist Hans Berger pioneered the mapping of human brain activity in 1924, researchers at Stanford University have designed two tiny brain-insertable sensors connected to a computer algorithm to help translate thoughts to words to help paralyzed people express themselves. On August 23, a study demonstrating the use of such a device on human patients was published in Nature. (A similar study was also published in Nature on the same day.)
What the researchers created is a brain-computer interface (BCI)—a system that translates neural activity to intended speech—that helps paralyzed individuals, such as those with brainstem strokes or amyotrophic lateral sclerosis (ALS), express their thoughts through a computer screen. Once implanted, pill-sized sensors can send electrical signals from the cerebral cortex, a part of the brain associated with memory, language, problem-solving and thought, to a custom-made AI algorithm that can then use that to predict intended speech.
This BCI learns to identify distinct patterns of neural activity associated with each of the 39 phonemes, or the smallest part of speech. These are sounds within the English language such as “qu” in quill, “ear” in near, or “m” in mat. As a patient attempts speech, these decoded phonemes are fed into a complex autocorrect program that assembles them into words and sentences reflective of their intended speech. Through ongoing practice sessions, the AI software progressively enhances its ability to interpret the user’s brain signals and accurately translate their speech intentions.
“The system has two components. The first is a neural network that decodes phonemes, or units of sound, from neural signals in real-time as the participant is attempting to speak,” says the study’s co-author Erin Michelle Kunz, an electrical engineering PhD student at Stanford University, via email. “The output sequence of phonemes from this network is then passed into a language model which turns it into text of words based on statistics in the English language.”
With 25, four-hour-long training sessions, Pat Bennett, who has ALS—a disease that attacks the nervous system impacting physical movement and function—would practice random samples of sentences chosen from a database. For example, the patient would try to say: “It’s only been that way in the last five years” or “I left right in the middle of it.” When Bennett, now 68, attempted to read a sentence provided, her brain activity would register to the implanted sensors, then the implants would send signals to an AI software through attached wires to an algorithm to decode the brain’s attempted speech with the list of phonemes, which would then be strung into words provided on the computer screen. The algorithm in essence acts as a phone’s autocorrect that kicks in during texting.
“This system is trained to know what words should come before other ones, and which phonemes make what words,” Willett said. “If some phonemes were wrongly interpreted, it can still take a good guess.”
By participating in twice-weekly software training sessions for almost half a year, Bennet was able to have her attempted speech translated at a rate of 62 words a minute, which is faster than previously recorded machine-based speech technology, says Kunz and her team. Initially, the vocabulary for the model was restricted to 50 words—for straightforward sentences such as “hello,” “I,” “am,” “hungry,” “family,” and “thirsty”—with a less than 10 percent error, which then expanded to 125,000 words with a little under 24 percent error rate.
While Willett explains this is not “an actual device people can use in everyday life,” but it is a step towards ramping up communication speed so speech-disabled persons can be more assimilated to everyday life.
“For individuals that suffer an injury or have ALS and lose their ability to speak, it can be devastating. This can affect their ability to work and maintain relationships with friends and family in addition to communicating basic care needs,” Kunz says. “Our goal with this work was aimed at improving quality of life for these individuals by giving them a more naturalistic way to communicate, at a rate comparable to typical conversation.”
Watch a brief video about the research, below:
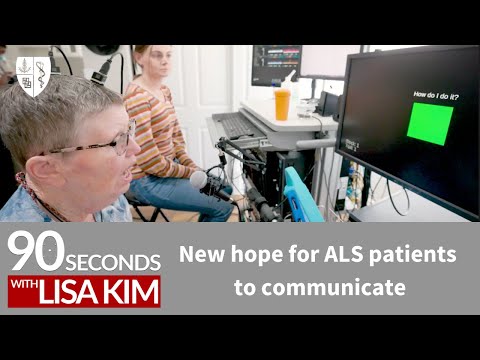