

Gina Ciavarra is sitting in a dark room at NYU Langone Health in Manhattan. It’s a reading room, a space for radiologists like her to examine X-ray and MRI scans. The monitors in front of her display grayscale images of a de-identified patient’s knee, and in them she detects one key problem: a torn ACL. “This is definitely abnormal,” Ciavarra explains.
But there’s another evaluation that Ciavarra must make, in addition to scanning the swirls of bone, ligaments, fat, cartilage, and tendons for problems like tears or arthritis. Was this particular knee scan created by artificial intelligence, or did it emerge from an MRI machine the traditional way? “My gut says it’s AI,” she says, without certainty. “It just looks a little blurry.”
Ciavarra and her NYU colleagues were participants in a study that pitted the quality of AI-created scans against traditional ones. By pairing artificial intelligence with MRI machines, computer scientists and radiologists think they can greatly speed up a common type of medical exam—a boon for patients and hospitals alike. That could mean cutting a ten-minute knee scan to five minutes, or an hour-long cardiac scan to half an hour. It could also save hospitals money, and reduce the need to anesthetize pediatric patients who may have trouble holding still.
The study, which NYU is now preparing to submit for academic review, is part of a project between two strange bedfellows: the NYU School of Medicine and Facebook. The partnership, initiated by the Facebook Artificial Intelligence Research division and announced over a year ago, has a simple goal: use AI to develop quick yet high-quality MRI scans that could someday allow busy medical centers to care for more people, countries with scant resources to make better use of the equipment they do have, and the elderly, young, and claustrophobic to spend less time in a narrow and loud magnetic tube.
The upshot of using AI in this way is that it requires a lot less information than the well-established approach (called an inverse Fourier transform) does when creating the images that give doctors an inside look at the human body. “In MRI we acquire a certain amount of data and then use reconstruction methods to create an image,” says Michael Recht, the chair of the radiology department at NYU Langone Health. “But it turns out we’ve always collected more data than we probably need.” Think of it like a fuel-efficient car replacing a gas-guzzling clunker: The new algorithm needs less data, from fewer measurements, to go the same distance (or in this case, get the right picture) as the MRI machine.
To get a radiologist or surgeon the intel they need—and for this experiment to be considered a success—an AI-generated image has to check two boxes, explains Larry Zitnick, a research scientist at FAIR. First, it has to be accurate: A pretty scan that misses a tear in a ligament or invents something that isn’t actually there can be both useless and dangerous. Second, “the radiologists have to like the image,” Zitnick says. When doctors like Ciavarra spend hours in dark reading rooms staring at scans, they need photos that are sharp and easy on the eyes.
Getting an algorithm to interpret the information that such a tried-and-tested machine produces is no easy task, though. To train the AI software to correctly spin frequency data into images, the Facebook team says they tried around 1,000 different model variations with information from real MRI scans. They gave the algorithm raw information, and also showed it the corresponding images to help the neural network (a common machine-learning tool that software engineers can train to perform different tasks, like recognize what’s in a photograph) generate the correct images.

Once Facebook developed the model, it had to blind test it on the eagle-eyed experts. NYU radiologists like Ciavarra reviewed knee scans spun into existence by AI and those made the old-fashioned way to see if they could get the same diagnostic information from both. They then had to guess which was which. Rather than scanning patients twice—the slower, regular way and the faster, AI-powered approach—the team retroactively stripped some of the raw data from regular scans to simulate what running the machine faster would have looked like.
Zitnick also notes that his crew added a little bit of noise to the AI-generated images to make them look more realistic and avoid tipping their hands to the doctors. “You tweak it just right, and then suddenly the radiologists have a really hard time telling which one is from the AI and which one isn’t, because you’re taking away that one hint that was there,” he says. (The added noise didn’t affect the diagnostic value of the scan, he says.)
Typically, when you hear about the intersection of AI and radiology, the algorithm is analyzing images, not creating them like in the Facebook-NYU project. “I think that this is a very exciting and important direction of study,” says Maciej Mazurowski, an associate professor at Duke University, who focuses on radiology and AI but isn’t involved in this MRI work. “It’s different from what most of the radiology AI studies are.” For example, Mazurowski has used a neural network to evaluate nodules on people’s thyroids in ultrasound scans. Other research has focused on employing machine learning to look for problems like tuberculosis in chest images.
Facebook says that it will make their AI-MRI algorithm publicly available, so that other researchers who wants to work on the goal of running machines faster and using artificial intelligence to interpret data into images can do so. “The impact of this in a clinic can be tremendous because MRI scanners are expensive and they’re often backed up,” Mazurowski says. There are some potential risks to injecting AI into the process, however. For one, an algorithm could invent an issue that isn’t actually there (an artifact). More importantly, Mazurowski says, the bigger concern is that it could overlook an actual problem, meaning that the radiologist never notices an ACL tear.

It’s a high-stakes project with potentially crucial returns: A surgeon may cut, or not cut, depending on the results of a scan. “It totally makes us nervous,” Zitnick says. “It is important to get these things right, and that’s why we’re doing this in a very methodical way.”
As the interchangeability study waits on academic review, NYU researchers are gearing up to conduct further comparisons to gauge whether AI-created images match what a surgeon actually sees when they perform an arthroscopy inside a knee. The goal for the future is not to just limit this technology to knees, but to also use it for other body parts, like MRIs of the brain, which currently require a whole lot of scan time.
Recht, of NYU, says that he hopes that quick AI scans will change the relationship that physicians and patients have with MRIs. “My dream,” he says, “is to have five-minute scan times for every joint.”
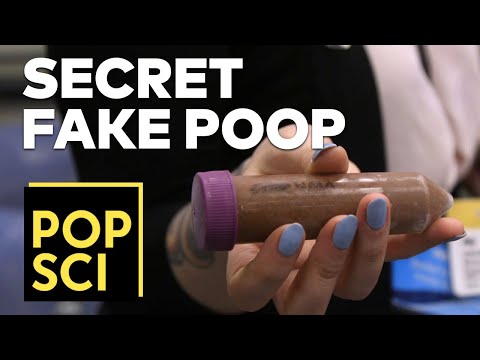