Here’s how Apple can figure out which emojis are popular
Welcome to the fascinating world of differential privacy.
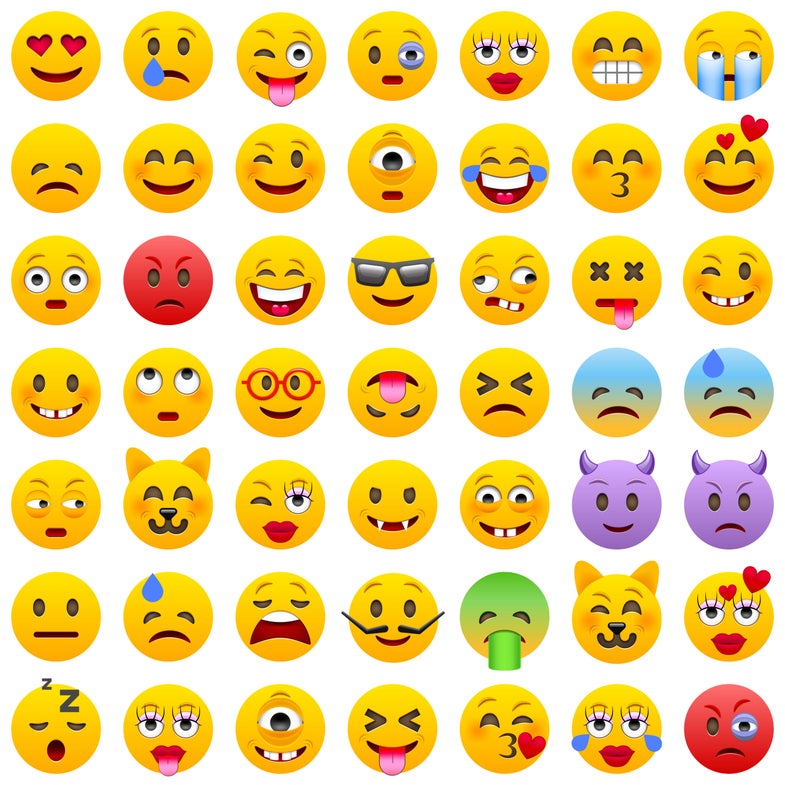
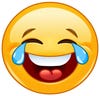
In a jargon-filled paper released by Apple, the company revealed a ranking of popular emojis its users send, and the big winner from that snapshot at least is the trusty old smiling face with tears of joy. The simple red heart is in second place.
Emojis are simple and silly, but the way that Apple figures out which emojis are popular is anything but. The company recently published the article with the emoji ranking in it on their Machine Learning Journal, and it explains how they gather big-picture data about stuff like emojis, while also protecting people’s privacy on an individual level.
To do that, they use a computer science strategy called differential privacy. In short, that means adding some sort of noise to obscure the data on a person’s phone, but later—after that noisy data is combined with other people’s noisy data—they can still understand what they’ve gathered on a big-picture level.
“Differential privacy” is a confusing term, but the concept is fascinating.
Imagine that you want to conduct a poll before an election to figure out what percentage of people are going to vote for the Democratic candidate, says Aaron Roth, an associate professor of computer and information science at the University of Pennsylvania. Pollsters call voters and ask them who they’re going to vote for, and record it in a ledger. But if that record were to be leaked or stolen, a whole list of people’s names and party preferences would be exposed. With this method, you know which candidate might win, but you’ve put people’s privacy at risk.
Now image that pollsters—who, like before, still want to know which candidate will likely win—call voters and ask them a different version of the question. It starts by asking a voter to flip a coin. If that coin turns up heads, the voter is instructed to tell the truth about which party he will vote for. But if it’s tails, he is told to choose randomly between the two parties and say one of them. In other words, tails means there’s a 50 percent chance the pollster hears Republican, and 50 percent Democrat. All told, using this method, there’s a 75 percent chance the pollster hear the truth out of the voter about who he will vote for, and a 25 percent chance they hear a falsehood. There’s noise, but that noise has been added deliberately. The pollsters don’t even know if the answer they are hearing is the true one or not, only the percentage chance that it is true.
What that means is that if the pollster’s ledger became public, no personal voter information would be compromised. “You wouldn’t be able to form strong beliefs about who any individual person was going to vote for,” Roth says. “Each individual would have plausible deniability.” If your data was leaked, no one would know if it was accurate or not.
But crucially, the pollsters can still calculate the average they need to predict the election, because they know the specific way they made the data noisy. The big picture is clear, but the small one is muddy.
“This is a very simple example,” Roth says, “but differential privacy provides a formal definition of privacy and a methodology for doing things like this more generally.”
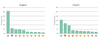
This is the general method Apple uses when figuring out trends about behavior like emoji use. “It is rooted in the idea that carefully calibrated noise can mask a user’s data,” the company writes on their machine learning blog. “When many people submit data, the noise that has been added averages out and meaningful information emerges.”
Differential privacy, Roth says, is an important tool when solving specific types of problems. If you’re trying to figure out if an individual has cancer and needs treatment, differential privacy is a bad strategy—obviously. But if you want to know what percentage of a certain population has cancer, differential privacy could be the way to figure that out. “Differential privacy is useful when the thing you want to learn about is actually not some fact about an individual, but some statistical property of a population,” Roth says.
Apple explains that when people opt into sharing this kind of data with them, after the noise is applied to the data on the phone, a random encrypted sampling of it goes to an Apple server. “These records do not include device identifiers or timestamps of when events were generated,” the company writes.
Any iOS user can choose whether to share or not: Go to Settings, then Privacy, then Analytics, and toggle “Share iPhone Analytics” off or on.