Here’s how an AI lie detector can tell when you’re fibbing
Deception detection meets artificial intelligence.
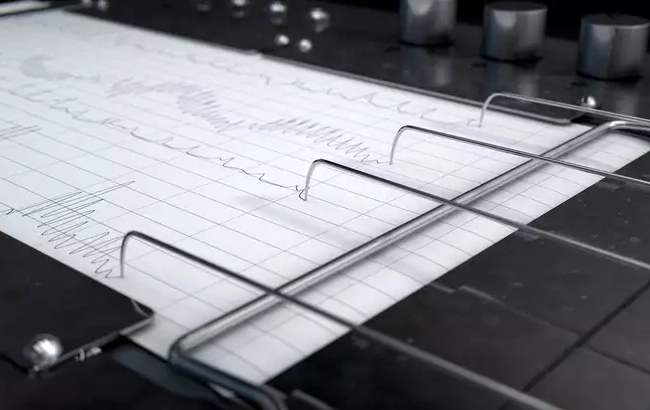
Artificial intelligence is everywhere—it figures out what’s in the food photos on sites like Yelp, it helps researchers attempt to make MRI scans faster, and it can even look for signs of depression in someone’s voice. But here’s a use you may not have considered: lie detection.
That idea—an AI fib sniffer—is in the news because of a border security project in Europe called iBorderCtrl that involves technology focused on “deception detection.” The initiative includes a two-step process, and the lie-detection part happens at home. According to the European Commission, the protocol begins with a pre-screening in which voyagers “use a webcam to answer questions from a computer-animated border guard, personalised to the traveller’s gender, ethnicity and language. The unique approach to ‘deception detection’ analyses the micro-expressions of travellers to figure out if the interviewee is lying.”
It sounds like science fiction, and of course, it also brings to mind the troubling history of polygraph tests. But such an AI system is possible. The question is: How accurate can it be?
Rada Mihalcea, a professor of computer science and engineering at the University of Michigan, has worked on deception detection for about a decade. This is how they constructed one AI deception detector, and how it works.
The first thing that researchers working on artificial intelligence and machine learning need is data. In the case of the work that Mihalcea did, they began with videos from actual court cases. For example, a defendant speaking in a trial in which they were found guilty could provide an example of deceit; they also used testimony from witnesses as either examples of truthful or deceitful statements. (Of course, machine learning algorithms are only as good as the data fed into it, and it is important to remember that someone found guilty of a crime may in fact be innocent.)
All told, they used 121 video clips and the corresponding transcripts of what they said—about half represented deceptive statements, and half truthful. It was this data that they used to build machine learning classifiers that ultimately had between a 60 to 75 percent accuracy rate.
One thing the system noticed? “The use of pronouns—people who are lying would tend to less often use the word ‘I’ or ‘we,’ or things that refer to themself,” Mihalcea explains. “Instead, people who are lying would more often use ‘you,’ ‘yours,’ ‘he,’ ‘they,’ [and] ‘she.’”
That’s not the only linguistic signal: someone telling a lie would use “stronger words” that “reflect certainty,” she says. Examples of those types of words are “absolutely,” and “very,” while interestingly, people telling the truth were more likely to hedge, using words such as “maybe” or “probably.”
“I think people who are deceptive would try to make up for the lie they are putting forward,” she says, “and so they try to seem more certain of themselves.”
As for gestures, she points out that someone being deceitful would more likely look directly into the eyes of the person questioning them. They also tended to use both hands when gesturing, instead of just one—also, she suspects, as part of trying to be convincing. (Of course, these are patterns she’s describing: if someone looks you in the eyes and gestures with both hands while speaking, it doesn’t mean they are lying.)
These are all the fascinating little data points that AI can begin to notice after researchers give it examples to work with and learn from. But Mihalcea’s work is “not perfect,” she concedes. “As a researcher, we are excited we were able to get to 75 percent [accuracy].” But looked at another way, that’s an error rate of one in four. “I don’t think it’s ready to be used in practice, because of the 25 percent error [rate].”
Ultimately, she sees technology like this as being assistive for people—it could, for example, indicate that it noticed something “unusual” in a speaker’s statement, and then perhaps have a person “probe more.” And that is actually a frequent use-case for AI: tech that augments what humans can do.