How video game tech, AI, and computer vision help decode animal pain and behavior
Top neuroscience labs are adapting new and unexpected tools to gain a deeper understanding of how mice, and ultimately humans, react to different drug treatments.
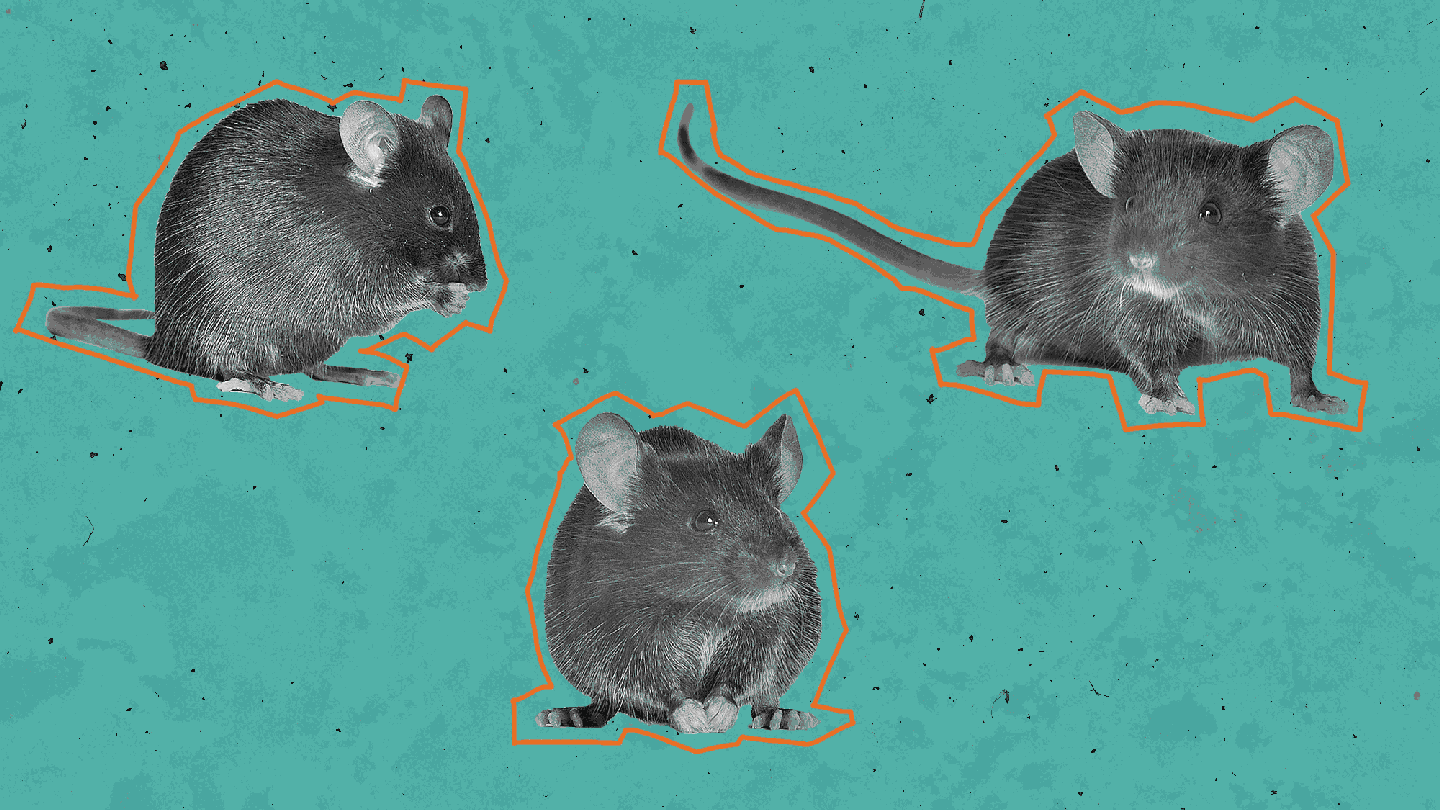
Back in 2013, Sandeep Robert “Bob” Datta was working in his neurobiology lab at Harvard Medical School in Boston when he made the fateful decision to send his student Alex Wiltschko to the Best Buy up the street. Wiltschko was on a mission to purchase an Xbox Kinect camera, designed to pick up players’ body movements for video games like Just Dance and FIFA. He plunked down about $150 and walked out with it. The unassuming piece of consumer electronics would determine the lab’s direction in the coming decade and beyond.
It also placed the team within a growing scientific movement at the intersection of artificial intelligence, neuroscience, and animal behavior—a field poised to change the way researchers use other creatures to study human health conditions. The Datta Lab is learning to track the intricate nuances of mouse movement and understand the basics of how the mammal brain creates behavior, untangling the neuroscience of different health conditions and ultimately developing new treatments for people. This area of research relies on so-called “computer vision” to analyze video footage of animals and detect behavior patterns imperceptible to the unaided eye. Computer vision can also be used to auto-detect cell types, addressing a persistent problem for researchers who study complex tissues in, for example, cancers and gut microbiomes.
In the early 2010s, Datta’s lab was interrogating how smell, “the sense that is most important to most animals” and the one that mice can’t survive without, drives the rodents’ responses to manipulations in their environment. Human observers traditionally track mouse behavior and record their observations—how many times a mouse freezes in fear, how often it rears up to explore its enclosure, how long it spends grooming, how many marbles it buries. Datta wanted to move beyond the movements visible to the unaided eye and use video cameras to track and compute whether a rodent avoids an odor (that of predator urine, for instance) or is attracted to it (like the smell of roses). The tools available at the time—overhead 2D cameras that tracked each animal as a single point—didn’t yield sufficiently detailed data.
“Even in an arena in the dark, where there’s no stimuli at all, [mice] just generate these incredible behavioral dynamics—none of which are being captured by, like, a dot bouncing around on the screen,” says Datta. So Wiltschko identified the Xbox Kinect camera as a potential solution. Soon after its introduction in 2010, people began hacking the hardware for science and entertainment purposes. It was fitting for Datta’s lab to use it to track mice: It can record in the dark using infrared light (mice move around much more when it’s darker) and can see in 3D when mounted overhead by measuring how far an object is from the sensor. This enabled Datta’s team to follow the subjects when they ran around, reared up, or hunkered down. As it analyzed its initial results, it realized that the Kinect camera recorded the animals’ movements with a richness that 2D cameras couldn’t capture.
“That got us thinking that if we could just somehow identify regularities in the data, we might be able to identify motifs or modules of action,” Datta says. Looking at the raw pixel counts from the Kinect sensor, even as compressed image files and without any sophisticated analysis, they began seeing these regularities. With or without an odor being introduced, every few hundred milliseconds, mice would switch between different types of movement—rearing, bobbing their heads, turning. For several years after the first Kinect tests, Datta and his team tried to develop software to identify and record the underlying elements of the basic components of movement the animals string together to create behavior.
But they kept hitting dead ends.
“There are many, many ways you can take data and divide it up into piles. And we tried many of those ways, many for years,” Datta recalls. “And we had many, many false starts.”
They tried categorizing results based on the animals’ poses from single frames of video, but that approach ignored movement—“the thing that makes behavior magic,” according to Datta. So they abandoned that strategy and started thinking about the smaller motions that last fractions of a second and constitute behavior, analyzing them in sequence. This was the key: the recognition that movement is both discrete and continuous, made up of units but also fluid.
So they started working with machine learning tools that would respect this dual identity. In 2020, seven years after that fateful trip to Best Buy, Datta’s lab published a scientific paper describing the resulting program, called MoSeq (short for “motion sequencing,” evoking the precision of genetic sequencing). In this paper, they demonstrated their technique could identify the subsecond movements, or “syllables,” as they call them, that make up mouse behavior when they’re strung together into sequences. By detecting when a mouse reared, paused, or darted away, the Kinect opened up new possibilities for decoding the “grammar” of animal behavior.
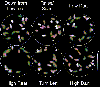
Computer visionaries
In the far corner of the Datta Lab, which still resides at Harvard Medical School, Ph.D. student Maya Jay pulls back a black curtain, revealing a small room bathed in soft reddish-orange light. To the right sit three identical assemblies made of black buckets nestled inside metal frames. Over each bucket hangs a Microsoft Xbox Kinect camera, as well as a fiber-optic cable connected to a laser light source used to manipulate brain activity. The depth-sensing function of the cameras is the crucial element at play. Whereas a typical digital video captures things like color, the images produced by the Kinect camera actually show the height of the animal off the floor, Jay says—for instance, when it bobs its head or rears up on its hind legs.
Microsoft discontinued the Xbox Kinect cameras in 2017 and has stopped supporting the gadget with software updates. But Datta’s lab developed its own software packages, so it doesn’t rely on Microsoft to keep the cameras running, Jay says. The lab also runs its own software for the Azure Kinect, a successor to the original Kinect that the team also employs—though it was also discontinued, in 2023. Across the lab from the Xbox Kinect rigs sits a six-camera Azure setup that records mice from all angles, including from below, to generate either highly precise 2D images incorporating data from various angles or 3D images.
In the case of MoSeq and other computer vision tools, motion recordings are often analyzed in conjunction with manipulations to the brain, where sensory and motor functions are rooted in distinct modules, and neural-activity readings. When disruptions in brain circuits, either from drugs administered in the lab or edits to genes that mice share with humans, lead to changes in behaviors, it suggests a connection between the two. This makes it possible for researchers to determine which circuits in the brain are associated with certain types of behavior, as well as how medications are working on these circuits.
In 2023, Datta’s lab published two papers detailing how MoSeq can contribute to new insights into an organism’s internal wiring. In one, the team found that, for at least some mice in some situations, differences in mouse behavior are influenced way more by individual variation in the brain circuits involved with exploration than by sex or reproductive cycles. In another, manipulating the neurotransmitter dopamine suggested that this chemical messenger associated with the brain’s reward system supports spontaneous behavior in much the same way it influences goal-directed behaviors. The idea is that little bits of dopamine are constantly being secreted to structure behavior, contrary to the popular perception of dopamine as a momentous reward. The researchers did not compare MoSeq to human observations, but it performed comparably in another set of experiments in a paper that has yet to be published.
These studies probed some basic principles of mouse neurobiology, but many experts in this field say MoSeq and similar tools could broadly revolutionize animal and human health research in the near future.
With computer vision tools, mouse behavioral tests can run in a fraction of the time that would be required with human observers. This tech comes at a time when multiple forces are calling animal testing into question. The United States Food and Drug Administration (FDA) recently changed its rules on drug testing to consider alternatives to animal testing as prerequisites for human clinical trials. Some experts, however, doubt that stand-ins such as organs on chips are advanced enough to replace model organisms yet. But the need exists. Beyond welfare and ethical concerns, the vast majority of clinical trials fail to show benefits in humans and sometimes produce dangerous and unforeseen side effects, even after promising tests on mice or other models. Proponents say computer vision tools could improve the quality of medical research and reduce the suffering of lab animals by detecting their discomfort in experimental conditions and clocking the effects of treatments with greater sensitivity than conventional observations.
Further fueling scientists’ excitement, some see computer vision tools as a means of measuring the effects of optogenetics and chemogenetics, techniques that use engineered molecules to make select brain cells turn on in response to light and chemicals, respectively. These biomedical approaches have revolutionized neuroscience in the past decade by enabling scientists to precisely manipulate brain circuits, in turn helping them investigate the specific networks and neurons involved in behavioral and cognitive processes. “This second wave of behavior quantification is the other half of the coin that everyone was missing,” says Greg Corder, assistant professor of psychiatry at the University of Pennsylvania. Others agree that these computer vision tools are the missing piece to track the effects of gene editing in the lab.
“[These technologies] truly are integrated and converge,” agrees Clifford Woolf, a neurobiologist at Harvard Medical School who works with his own supervised computer vision tools in his pain research.
Adult supervision recommended
But is artificial intelligence ready to take over the task of tracking animal behavior and interpreting its meaning? And is it identifying meaningful connections between behavior and neurological activity just yet?
These are the questions at the heart of a tension between supervised and unsupervised AI models. Machine learning algorithms find patterns in data at speeds and scales that would be difficult or impossible for humans. Unsupervised machine learning algorithms identify any and all motifs in datasets, whereas supervised ones are trained by humans to identify specific categories. In mouse terms, this means unsupervised AIs will flag every unique movement or behavior, but supervised ones will pinpoint only those that researchers are interested in.
The major advantage of unsupervised approaches for mouse research is that people may not notice action that takes place on the subsecond scale. “When we analyze behavior types, we often actually are based on the experimenters’ judgment of the behavior type, rather than mathematical clustering,” says Bing Ye, a neuroscientist at the University of Michigan whose team developed LabGym, a supervised machine learning tool for mice and other animals, including rats and fruit fly larvae. The number of behavioral clusters that can be analyzed, too, is limited by human trainers. On the other hand, he says, live experts may be the most qualified to recognize behaviors of note. For this reason, he advocates transparency: publishing training datasets, the classification parameters that a supervised algorithm learns on, with any studies. That way, if experts disagree with how a tool identifies behaviors, the publicly available data provide a solid foundation for scientific debate.
Mu Yang, a neurobiologist at Columbia University and the director of the Mouse NeuroBehavior Core, a mouse behavior testing facility, is wary of trusting AI to do the work of humans until the machines have proved reliable. She is a traditional mouse behavior expert, trained to detect the animals’ subtleties with her own eyes. Yang knows that the way a rodent expresses an internal state, like fear, can change depending on its context. This is true for humans too. “Whether you’re in your house or…in a dark alley in a strange city, your fear behavior will look different,” Yang explains. In other words, a mouse may simply pause or it may freeze in fear, but an AI could be hard-pressed to tell the difference. One of the other challenges in tracking the animals’ behaviors, she says, is that testing different drugs on them may cause them to exhibit actions that are not seen in nature. Before AIs can be trusted to track these novel behaviors or movements, machine learning programs like MoSeq need to be vetted to ensure they can reliably track good old-fashioned mouse behaviors like grooming.
Yang draws a comparison to a chef, saying that you can’t win a Michelin star if you haven’t proved yourself as a short-order diner cook. “If I haven’t seen you making eggs and pancakes, you can talk about caviar and Kobe beef all you want, I still don’t know if I trust you to do that.”
For now, as to whether MoSeq can make eggs and pancakes, “I don’t know how you’d know,” Datta says. “We’ve articulated some standards that we think are useful. MoSeq meets those benchmarks.”
Putting the tech to the test
There are a couple of ways, Datta says, to determine benchmarks—measures of whether an unsupervised AI is correctly or usefully describing animal behavior. “One is by asking whether or not the content of the behavioral description that you get [from AI] does better or worse at allowing you to discriminate among [different] patterns of behavior that you know should occur.” His team did this in the first big MoSeq study: It gave mice different medicines and used the drugs’ expected effects to determine whether MoSeq was capturing them. But that’s a pretty low bar, Datta admits—a starting point. “There are very few behavioral characterization methods that wouldn’t be able to tell a mouse on high-dose amphetamine from a control.”
The real benchmark of these tools, he says, will be whether they can provide insight into how a mouse’s brain organizes behavior. To put it another way, the scientifically useful descriptions of behavior will predict something about what’s happening in the brain.
Explainability, the idea that machine learning will identify behaviors experts can link to expected behaviors, is a big advantage of supervised algorithms, says Vivek Kumar, associate professor at the biomedical research nonprofit Jackson Laboratory, one of the main suppliers of lab mice. His team used this approach, but he sees training supervised classifiers after unsupervised learning as a good compromise. The unsupervised learning can reveal elements that human observers may miss, and then supervised classifiers can take advantage of human judgment and knowledge to make sure that what an algorithm identifies is actually meaningful.
“It’s not magic”
MoSeq isn’t the first or only computer vision tool under development for quantifying animal behavior. In fact, the field is booming as AI tools become more powerful and easier to use. We already mentioned Bing Ye and LabGym; the lab of Eric Yttri at Carnegie Mellon University has developed B-SOiD; the lab of Mackenzie Mathis at École Polytechnique Fédérale de Lausanne has DeepLabCut; and the Jackson Laboratory is developing (and has patented) its own computer vision tools. Last year Kumar and his colleagues used machine vision to develop a frailty index for mice, an assessment that is notoriously sensitive to human error.
Each of these automated systems has proved powerful in its own way. For example, B-SOiD, which is unsupervised, identified the three main types of mouse grooming without being trained in these basic behaviors.
“That’s probably a good benchmark,” Yang says. “I guess you can say, like the egg and pancake.”
Mathis, who developed DeepLabCut, emphasizes that carefully picking data sources is critical for making the most of these tools. “It’s not magic,” she says. “It can make mistakes, and your trained neural networks are only as good as the data you give [them].”
And while the toolmakers are still honing their technologies, even more labs are hard at work deploying them in mouse research with specific questions and targets in mind. Broadly, the long-term goal is to aid in the discovery of drugs that will treat psychiatric and neurological conditions.
Some have already experienced vast improvements in running their experiments. One of the problems of traditional mouse research is that animals are put through unnatural tasks like running mazes and taking object recognition tests that “ignore the intrinsic richness” of behavior, says Cheng Li, professor of anesthesiology at Tongji University in Shanghai. His team found that feeding MoSeq videos of spontaneous rodent behavior along with more traditional task-oriented behaviors yielded a detailed description of the mouse version of postoperative delirium, the most common central nervous system surgical complication among elderly people.
Meanwhile, LabGym is being used to study sudden unexpected death in epilepsy in the lab of Bill Nobis at Vanderbilt University Medical Center. After being trained on videos of mouse seizures, the program detects them “every time,” Nobis says.
Easing their pain
Computer vision has also become a major instrument for pain research, helping to untangle the brain’s pathways involved in different types of pain and treat human ailments with new or existing drugs. And despite the FDA rule change in early 2023, the total elimination of animal testing is unlikely, Woolf says, especially in developing novel medicines. By detecting subtle behavioral signs of pain, computer vision tools stand to reduce animal suffering. “We can monitor the changes in them and ensure that we’re not producing an overwhelming, painful situation—all we want is enough pain that we can measure it,” he explains. “We would not do anything to a mouse that we wouldn’t do to a human, in general.”
His team used supervised machine learning to track behavioral signatures of pain in mice and show when medications have alleviated their discomfort, according to a 2022 paper in the journal Pain. One of the problems with measuring pain in lab animals, rather than humans, is that the creatures can’t report their level of suffering, Woolf says. Scientists long believed that, proportional to body weight, the amount of medicine required to relieve pain is much higher in mice than in humans. But it turns out that if your computer vision algorithms can measure the sensation relatively accurately—and Woolf says his team’s can—then you actually detect signs of pain relief at much more comparable doses, potentially reducing the level of pain inflicted to conduct this research. Measuring pain and assessing pain medicine in lab animals is so challenging that most large pharmaceutical companies have abandoned the area as too risky and expensive, he adds. “We hope this new approach is going to bring them back in.”
Corder’s lab at the University of Pennsylvania is working on pain too, but using the unsupervised B-SOiD in conjunction with DeepLabCut. In unpublished work, the team had DeepLabCut visualize mice as skeletal stick figures, then had B-SOiD identify 13 different pain-related behaviors like licking or biting limbs. Supervised machine learning will help make his team’s work more reliable, Corder says, as B-SOiD needs instruction to differentiate these behaviors from, say, genital licking, a routine hygiene behavior. (Yttri, the co-creator of B-SOiD, says supervision will be part of the new version of his software.)
As computer vision tools continue to evolve, they could even help reduce the number of animals required for research, says FDA spokesperson Lauren-Jei McCarthy. “The agency is very much aligned with efforts to replace, reduce, or refine animal studies through the use of appropriately validated technologies.”
If you build it, they will come
MoSeq’s next upgrade, which has been submitted to an academic journal and is under review, will try something similar to what Corder’s lab did: It will meld its unsupervised approach with keypoint detection, a computer vision method that highlights crucial points in an object like the body of a mouse. This particular approach employs the rig of six Kinect Azure cameras instead of the Datta lab’s classic Xbox Kinect camera rigs.
An advantage of this approach, Datta says, is that it can be applied to existing 2D video, meaning that all the petabytes of archival mouse data from past experiments could be opened up to analysis without the cost of running new experiments on mice. “That would be huge,” Corder agrees.
Datta’s certainty increases as he rattles off some of his team’s accomplishments with AI and mouse behavior in the past few years. “Can we use MoSeq to identify genetic mutants and distinguish them from wild types? —mice with genetics as they appear in nature. This was the subject of a 2020 paper in Nature Neuroscience, which showed that the algorithm can accurately discern mice with an autism-linked gene mutation from those with typical genetics. “Can we make predictions about neural activity?” The Datta Lab checked this off its bucket list just this year in its dopamine study. Abandoning the hedging so typical of scientists, he confidently declares, “All of that is true. I think in this sense, MoSeq can make eggs and pancakes.”