Google is working on ‘self-healing’ maps, thanks to artificial intelligence
What’s better than making a map? Making a machine that makes maps.
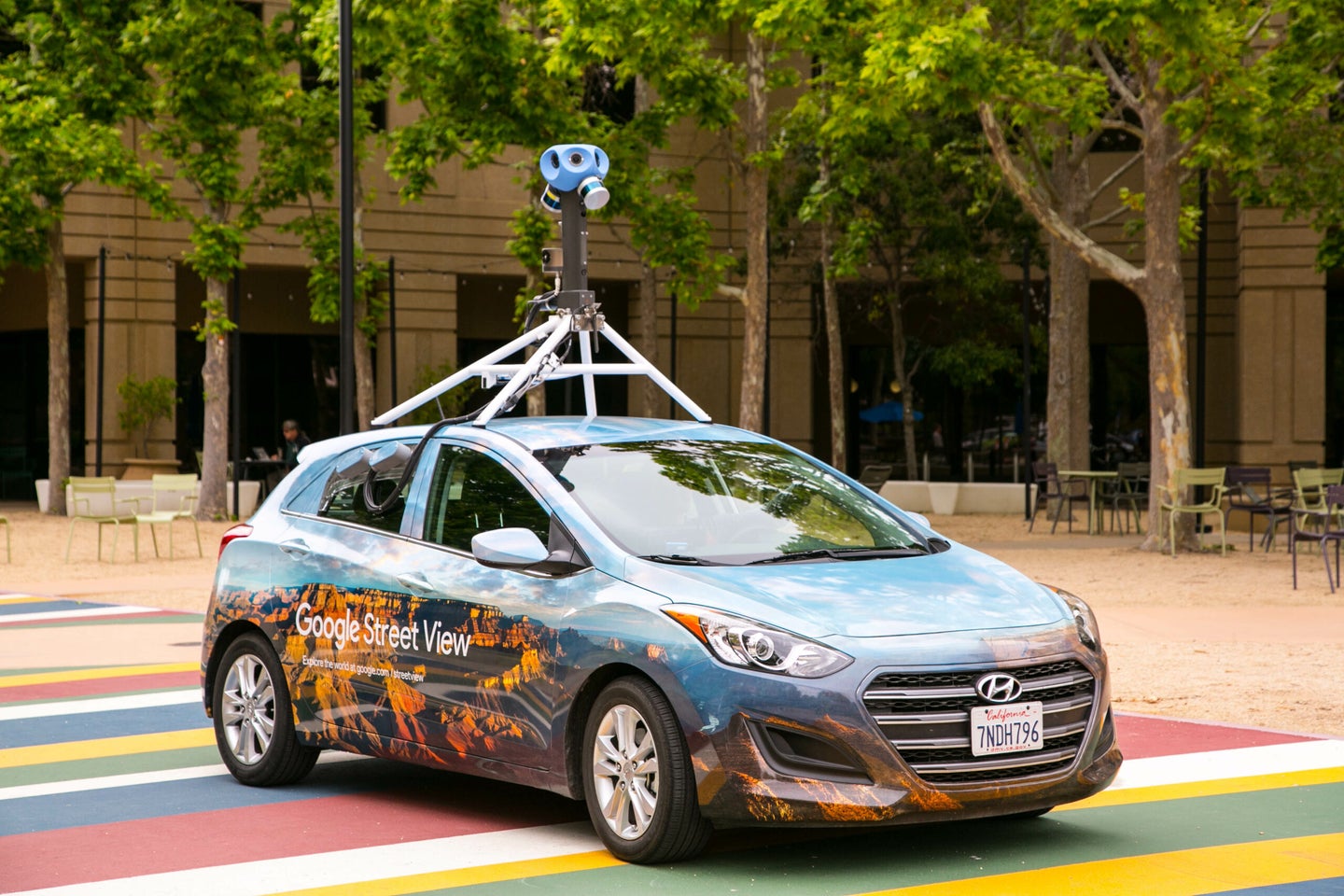
Zoom in on the Google Maps app on your phone, and eventually the shapes of buildings will come into view. You can thank artificial intelligence for helping to create scenes like that one—and that’s not all AI is doing for the app. Over recent years, the company has turned more to machine learning to automatically keep tabs on the world’s changing geography and then update how that’s reflected cartographically.
In fact, Google hit an inflection point around 2015 when it realized it had to change its strategy for keeping their maps updated, according to two Google Maps staffers who spoke exclusively with Popular Science. Andrew Lookingbill, the director of engineering for Google Maps, describes the moment as an “epiphany.”
Keeping maps updated in over 200 countries is hard—so the team had to pivot from just making maps to something more meta. “We needed to start making the machine that makes the map,” Lookingbill explains.
The way this is happening is through machine learning algorithms that are good enough to take imagery—like the pictures produced by those street view cars, or from satellites—extract the information they need from them, and then update the map. That information is likely data such as the name of a road, a house number, or the shape of a building seen from above. Google has boasted about this topic before: a 2017 blog post describes their efforts creating an algorithm that can read street names in France and mentions that algorithms like that could update addresses on the map.
Imagine that someone builds a new house, and a street view car cruises by. “That may end up being searchable in our maps without a human ever being in the inner loop, or having to do anything with that,” adds Lookingbill. That process—of AI analyzing imagery and updating the map—is what he calls “the first step towards our maps becoming self-healing.”
Creating building outlines is one task, he says, where better AI has greatly sped things up. A machine learning algorithm can look at satellite imagery and then draw the shape of the building on the Google map. Thanks to that, “we were able to double the number of buildings we’ve got modeled worldwide,” Lookingbill says. That happened over the course of a year. “For a sense of scale,” he adds, “all of the previous buildings we’d had, had taken us a decade to map.” Google touches on this in a blog item it published today, which describes the way a previous algorithm created building outlines as looking “fuzzy” (the post also explains the general steps and data sources that go into their map-making).
Other work, still in its “nascent” stages, involves using AI to create new roads on the map from imagery it analyzes. That “road synthesis,” Lookingbill says, involves them “actually trying to figure out the geometry of roads that we don’t have already on the map, based on imagery.”
For the artificial intelligence algorithms to do things like create building outlines or map new roads, it’s using imagery like top-down satellite data; for extracting information like street names, house numbers, and business names, the company is relying on street view.
Of course, Google Maps is not the only game in town: we noted in June that Apple is creating maps with greater detail in its own app, and that you should see changes in the experience when you update to iOS 13 this fall.
And on a larger note, machine learning algorithms that train on data to then accomplish tasks, sometimes at a superhuman level, are common in the tech world. That can involve something mundane, such as Yelp using AI to analyze and organize the pizza and taco pics that its users upload. And AI doesn’t just do things like recognize what’s in images: it can also do a myriad of other things, like playing and winning games, whether it’s poker, or even a Rubik’s cube.