How to Predict Tomorrow’s Hit Songs Today
The next big thing in music was typically predicted by a talent scout’s “gut” reaction. Now they may have some competition from an unlikely source
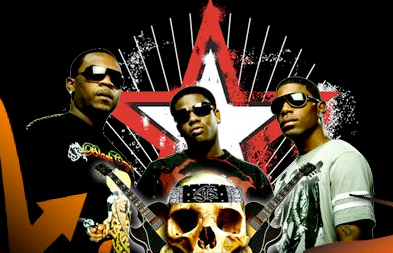
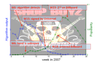
Charting a Winner
Researchers at Tel Aviv University’s School of Electrical Engineering have developed an algorithm that predicts the next big music superstar, and the accuracy is amazingly high: so far, it has a success rate of about 30 to 50 percent, according to lead researcher Yuval Shavitt.
The algorithm pulls data from Gnutella, which gets about 30 to 40 million queries a day and is currently the most popular peer-to-peer file-sharing network in the United States.
So far, the algorithm successfully predicted recent breakout artists Soulja Boy and Sean Kingston a full two months before they climbed to the top of Billboard charts in 2007. And when Atlanta-based rap trio Shop Boyz catapulted to fame in 2007, the algorithm predicted that, too—nine weeks before the group signed with Universal.
Shavitt’s algorithm could help record companies and music producers get early artist recommendations, and may have broader entertainment business applications, including predicting hit TV shows and YouTube videos. According to Shavitt, it could also be “applied to other electronically documented human activity” like online political forums, a subject he said might be a candidate for future research.
Location is key
While other methods have also successfully predicted a star’s rise (Soulja Boy, in particular), Shavitt’s algorithm is the first to focus on an artist’s popularity based on geography, a technique that he says he borrowed from the marketing world.
“It’s been known in marketing for several decades that the clustering of sales of a product indicate its success,” he said, “We used a similar idea, but the mathematic theory behind it is different since in our case there is typically only a single location where the young artist (which is like a new product) becomes popular.”
During a nine month period in 2007, Shavitt and his team analyzed both the query strings and geographic locations of Gnutella users to see what they were looking for and where they were looking from. Next, the algorithm identified queries that grew a substantial amount in a short period of time.
Interestingly enough, the breakout artists had huge local followings, even if they had no queries coming from anywhere else in the US. Exponential growth from a specific geographical region was the most reliable prediction for success. In some cases, the local following was constrained to a single neighborhood. But, Shavitt said, while the algorithm could be applied to a number of genres and locations, better predictions will come from popular music genres from areas with sufficient geographical spread.
More predictions
Shavitt’s group hopes to expand their predictions for the music industry. The next step, he said, is to find an algorithm to predict how long a song will remain at the top of the charts. His team is also looking at cultural differences between countries to see if the algorithms apply worldwide.
But while his predicting tool might impact how the music world finds its stars, Shavitt doesn’t see it as fully replacing the gut reaction of the talent scout.
“I see this as a tool that will save time and efforts to the human scouts. In general any technological advancement may make a few professions less required or even obsolete, but it opens the door for new professions. ‘Talking movies’ made the movie theater orchestras obsolete – but musicians found other ways to make a living,” he said.