Google’s A.I. Is Training Itself to Count Calories In Food Photos
Deep dish learning
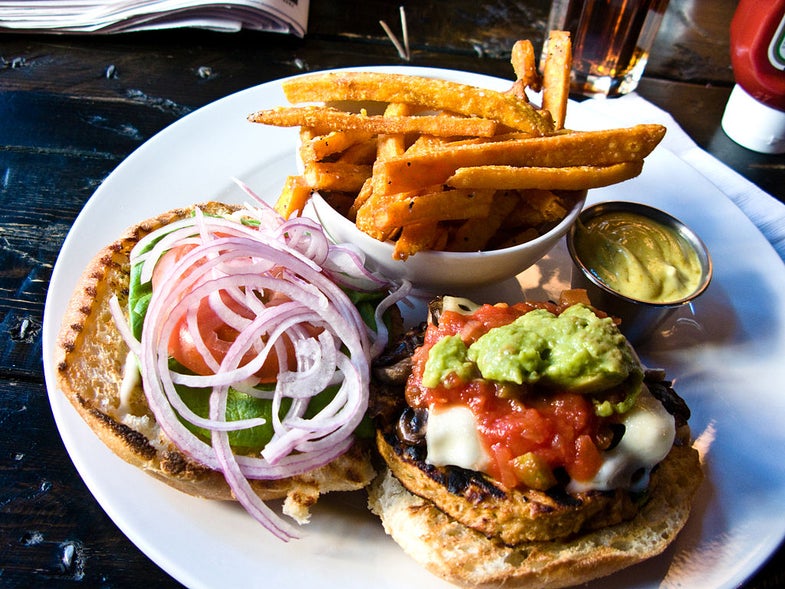
Whether by accident or design, the details of Google’s plans for artificial intelligence (AI) have been elusive. In some cases, there’s no real mystery, just nothing all that exciting to talk about. AI technology is the foundation of the company’s search engine, and the most obvious reason for Google’s high-profile, $400M acquisition of DeepMind in 2014 is to use the UK firm’s expertise in deep learning—a subset of AI research, but more on that later—to bolster that core capability. But the Googleplex has absorbed other bright minds from the field of AI, as well as some of the most buzzed-about companies in robotics, with only some of that collective braintrust officially allocated to driverless cars, delivery drones or other publicly announced robotics or AI-related projects. What, exactly, are Google’s AI experts up to?
In a word: food.
At this week’s Rework Deep Learning Summit in Boston, Google research scientist Kevin Murphy unveiled a project that uses sophisticated deep learning algorithms to analyze a still photo of food, and estimate how many calories are on the plate. It’s called Im2Calories, and in one example, the system looked at an image, and counted two eggs, two pancakes and three strips of bacon. Since those aren’t exactly universal units of measurement, the system gauged the size of each piece of food, in relation to the plate, as well as any condiments. And Im2Calories doesn’t require carefully captured high-res images. Any standard Instagram-quality shot should do.
So what was the final calorie count? I was too busy scribbling down other numbers from that particular presentation slide to catch it. And the point of Im2Calories isn’t to shame users with its shocking calculations of their daily food intake. Murphy wants to simply the process of keeping a food diary, identifying foods so you don’t have to manually plug them into an app, and taking the guesswork out of nagging variables such as serving sizes. “We semi-automate,” Murphy said during his presentation, noting that you can correct the software using dropdowns, if it confuses fried eggs for poached, or misreads something entirely. “If it only works 30 percent of the time, it’s enough that people will start using it, we’ll collect data, and it’ll get better over time,” said Murphy.
Though obesity remains a crisis in the United States, and a commercial version of Im2Calories would probably be hugely popular, it’s how this system work that’s worth a closer look. Like many deep learning applications, it marries visual analysis—in this case, determining the depth of each pixel in an image—with pattern recognition. Im2Calories can draw connections between what a given piece of food looks like, and vast amounts of available caloric data. And while it’s best not to read too much into the term “deep learning,” one of those evocative AI word choices that’s practically daring non-researchers to panic, Im2Calories is designed to improve itself through use. The purpose of many deep learning systems is to minimize the amount of time spent feeding or quizzing a piece of software, to improve its performance. If Im2Calories spots a burger, it’s because the pixels in the image resemble those in existing shots of burgers, not because a researcher held the system’s hand, so to speak, during various practice runs. For deep learning to make itself useful, primarily by extracting meaning from audio, video, still imagery and text, it has to be at least somewhat self reliant.
And even if Im2Calories is never completely accurate, Murphy thinks it will have an impact. “To me it’s obvious that people really want this and this is really useful,” he said. “Ok fine, maybe we get the calories off by 20 percent. It doesn’t matter. We’re going to average over a week or a month or a year. And now we can start to potentially join information from multiple people and start to do population level statistics. I have colleagues in epidemiology and public health, and they really want this stuff.”
Google only recently filed for a patent for Im2Calories, and Murphy wouldn’t share details about when it might be available. But the long-term goal for this technology is more wide-reaching. And, frankly, a more obvious fit for Google. “If we can do this for food, that’s just the killer app,” Murphy said. “Suppose we did street scene analysis. We don’t want to just say there are cars in this intersection. That’s boring. We want to do things like localize cars, count the cars, get attributes of the cars, which way are they facing. Then we can do things like traffic scene analysis, predict where the most likely parking spot is. And since this is all learned from data, the technology is the same, you just change the data.”
Obesity is a scourge, and deserves all the sophisticated deployment of semantic image segmentation and deep neural networks that Google can muster. But robot cars that instinctively know which block is most likely to have a free parking spot, ten minutes from now? It’s not surprising that deep learning is drawing so much interest from Silicon Valley. If anything, it’s a surprise that it’s taken this long.