Collaborative Algorithm Lets Autonomous Robots Team Up And Learn From Each Other
Last week, MIT announced an exciting, but somewhat obscure breakthrough—a new algorithm, called AMPS, that turns teams of robots into...
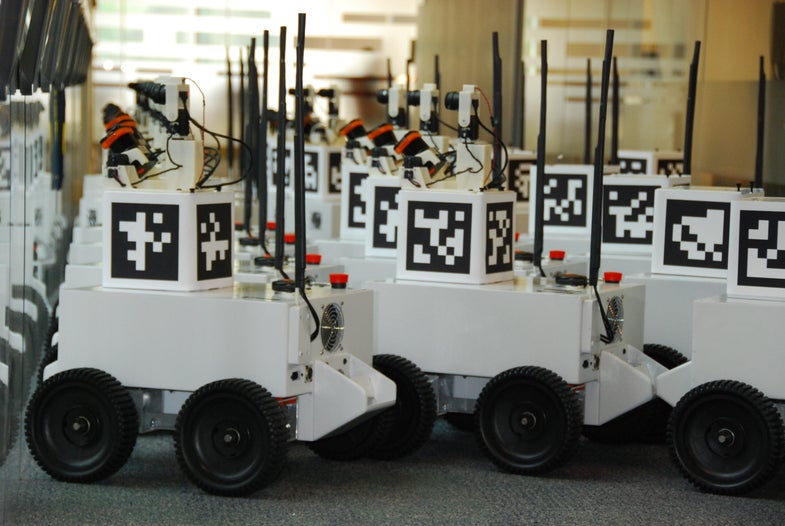
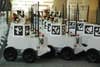
Last week, MIT announced an exciting, but somewhat obscure breakthrough—a new algorithm, called AMPS, that turns teams of robots into better learners. It lets autonomous systems quickly compare notes about what they’ve observed in their respective travels, and come up with a combined worldview.
If it seems like I’ve already succumbed to the worst temptations of robotics coverage—the urge to anthropomorphize machines, and to puree a discrete research achievement into a more easily digestible, broadly accessible slurry—bear with me. Though its authors aren’t calling it a breakthrough, this algorithm appears to be just that.
AMPS, which is short for Approximate Merging of Posteriors for Symmetry (a reference to Bayesian statistical analysis), will be presented at the Conference on Uncertainty in Artificial Intelligence in July. The algorithm tackles an extremely specific robotics problem. For a machine to operate in a given environment, it needs to assign semantic labels wherever possible. These are, in effect, cognitive shortcuts. So a rectangular section of the wall with hinges and a handle isn’t always a puzzle, to be solved from scratch every time it’s encountered. It’s a door, which can be opened or closed. And sets of semantic labels can add up to bigger labels. A door (label) that opens up onto a room with a large central table (another label) and a bunch of chairs (more labels), might be a conference room.
This sort of rampant labeling is as important to autonomous bots as it is to humans. The difference, however, is that people are generally more limber with their label creation and recognition. “We as humans tend to have a fairly well-defined vocabulary for what things are,” says Jonathan How, a professor of aeronautics and astronautics at MIT. “We know how to label things in a consistent global manner, or to pick them up by reading other things in our environment.” So if a person enters a conference room with no chairs in it, he or she doesn’t suddenly feel adrift in time and space. We’re smart like that.
Robots, comparatively speaking, can be rather dumb. Or rigid, at the very least. A chair-less conference room could be mistaken for a storage room, and forever labelled as such, long after the birthday party is over and the seats are returned. Far from anthropomorphizing them, this cognitive inflexibility is a reminder of just how inhuman robots are. And more problems can arise when machines try to share datasets, and combine their experiences into a larger collection of environmental labels. If one bot has registered an area as a conference room, and the other bot has labeled it as a storage room, how do they reconcile the discrepancy? Where humans could sort through the disagreement using our big mouths and still bigger brains, robots are stuck with their dueling, intransigent labels.
The AMPS algorithm promises to break these deadlocks, by allowing robots to reconsider the importance of various labels. “It’s more than just where things are, it’s what they are, what they’re composed of,” says How. For example, how crucial is it for a conference room to have chairs? And if one robot has already spotted what it considers a storage room, complete with boxes, cabinets and shelves, would there really be another storage room so close to it (without any of those tell-tale features)? According to How, who created the algorithm with his graduate student, Trevor Campbell, the trick is to allow the interfacing machines to establish new priorities for their labels, rebuilding their worldview. By allowing for conference rooms that may or may not have chairs in them, and reordering their labels to account for different experiences, the robots can achieve what How and Campbell refer to as semantic symmetry.
This is a solution to a problem that, to be honest, isn’t much of a problem yet. Autonomous systems are relatively rare outside of the well-defined, carefully labeled confines of manufacturing facilities, and ones that are designed to learn are rarer still. But as self-guided robots become more commonplace, and the environments and behaviors they have to navigate are more diverse, collaborative learning could be a serious asset. “It’s about building robots that aren’t constantly throwing their hands up in the air, saying, This isn’t one of the end things you defined. I don’t know what to do now,” says How.
AMPS, in other words, is for future generations of autonomous machines, such as robot cars, who will inevitably find themselves in situations that programmers didn’t have the foresight or bandwidth to prepare them for. Some cities, for example, can become a jaywalking free-for-all when the sun sets, forcing vehicles to creep through a steady flow of emboldened humans. A sheltered, suburban robot car that’s only seen pedestrians waiting patiently at crosswalks might do what robots so often do in novel, inexplicable situations, and grind to a halt. Meanwhile, a more city-based driverless vehicle may have more experience with this nightly quagmire of casual daredevilry and low-speed risk assessment. If these two bots stop at the same traffic light, and are able to effectively share their data, they might reconcile their disparate observations. The suburban model could break out of its stupor (or avoid falling into one in the first place) and proceed with a sufficient mix of caution and determination. The city slicker robot isn’t necessarily benefiting from learning about how humans behave in places where car culture reigns supreme, but maybe it picks up a trick or two related to blind driveways or rogues barreling down the breakdown lane.
Collaborative learning could be accomplished by other means, such as hooking machines up to an extensive, always-on network, where entire server farms can churn through clashing labels and update robots as needed. And the RoboEarth project, a self-described “Wikipedia for robots,” hopes to establish a universal knowledge base for bots to access. But AMPS’ advantage is its ability to work where constant network access isn’t an option, whether that means a gravel road in the Australian outback, or a crater gouged out of the surface of Mars. This approach focuses on robot-to-robot communication, without the luxury of powerful back-end systems. It essentially increases the autonomy of autonomous machines, and creates a foundation for meaningful learning. “We’re thinking about it this in the context of lifelong learning,” says How. “That means a robot could be out somewhere for a year operating on its own, and it doesn’t have to keep coming back and asking questions. Robots could roam around, just like people do, interacting individually or in pairs, finding ways to learn from each other.”
It’s far too early to know whether the AMPS algorithm will make its way into autonomous cars. But, as How points out, driverless vehicles are one of the main concerns at the Laboratory for Information and Decision Systems (the MIT research center that he’s affiliated with). A more short-term application might be in exploration or observation-based robots. Considering that this project was funded by the Office of Naval Research, a military system with a knack for teamwork seems entirely feasible. In the long run, though, collaborative learning is bigger than any single class of robot. It’s promise is the creation of more self-reliant bots, who don’t have to be walked through every task, and spoon-fed every relevant piece of data. Because if we surrender to the urge to anthropomorphize robots—and it’s hard not to—the autonomous ones are barely on their feet, and only occasionally out of diapers.